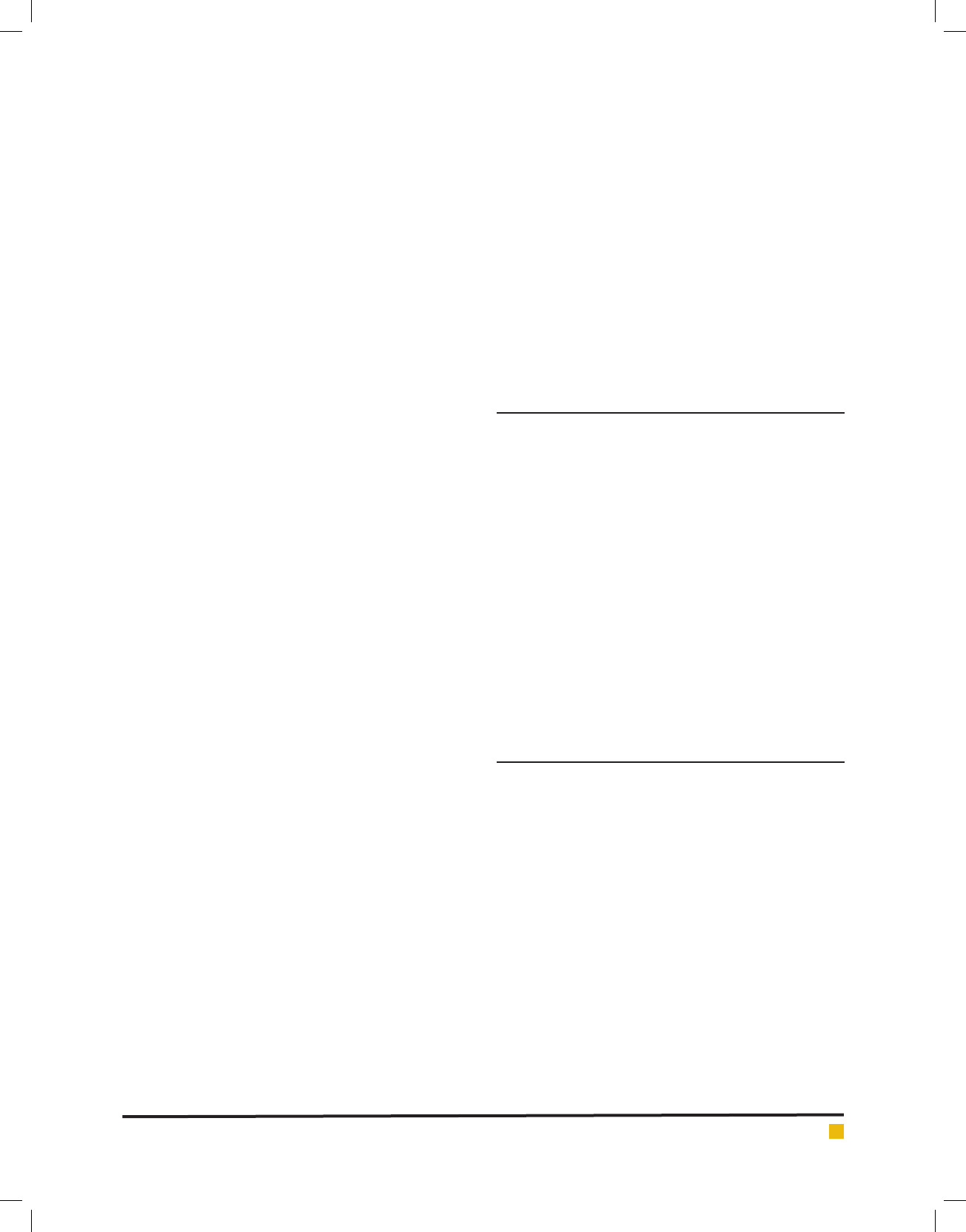
Archana and Lakshmi
BIOSCIENCE BIOTECHNOLOGY RESEARCH COMMUNICATIONS STATISTICAL OPTIMIZATION OF EXTRACELLULAR TANNASE PRODUCTION 697
ni cance of each of the coef cients and is indicative
of interaction strength of each independent variable.
P-value less than 0.05 indicate that the model terms are
signi cant. In this experiment, the Model F-value of
285.87 implied that the model is signi cant in enhanc-
ing enzyme production In this case A (Tannic acid ), G
(Dummy), H (Dummy) and J (Dummy) gave signi cant
terms and B, C, D, K and E gave negative signi cant
terms.
Optimization of signi cant variables by Response
surface methodology (RSM)
Tannic acid was found to be signi cant by PBD was
optimized by RSM using Box-Behnken design for tan-
nase production. The response values in terms of tan-
nase activity and the matrix design were represented in
Table 4.
The results obtained by Box-Behnken were analyzed
by Analysis of variance (ANOVA) with a model F-value
of 187.04 indicating that the model was signi cant
(Table 5). Values of Prob > F less than 0.05 indicate that
the model terms are signi cant. In the present model
A, AB, BC, AC, A
2
, B
2
are signi cant model terms. The
Model F-value of 187.04 implies the model is signi -
cant. There is only a 0.06% chance that an F-value this
large could occur due to noise. The Values of “Prob >
F” less than 0.0500 indicate model terms are signi -
cant.This t of the model was checked with the coef-
cient of determination R
2
, which was calculated to be
0.9680. The determination coef cient (R
2
) of the model
was 0.9980 indicating that 99.80% of variability in the
response could be accounted by the model.
The ANOVA gave the following regression equation:
R1 = +19.97 -3.28* A -0.24* B+0.30* C-1.82*
AB-1.24* AC+2.82* BC-6.79* A
2
-5.22*+0.000* C
2
Where R1 is the tannase activity; A is tannic acid; B is
KCl; C is KH
2
PO
4
and the interactions AB, BC, AC, A
2
, B
2
are signi cant.
3D Surface Plots
The three-dimensional response surface curves were
plotted to study the interaction among different phys-
icochemical factors used and to nd out the optimum
concentration of each factor for maximum tannase pro-
duction.
For the construction of 3D plots, effect of two vari-
ables was considered while the other variable was held
at zero. These plots were helpful in understanding both
the individual and the interaction effects of the two fac-
tors. For the construction of 3Dplots, effect of two vari-
ables was considered while the other variable was held
at zero. From the 3D plots, it was clear that the tannase
activity signi cantly increased with increase in the con-
centration of tannic acid (A) reaching to its maximum
at 18.12 U/ml/min, where as the enzyme activity gradu-
ally increased with increase KCl (B) concentration and
then declined (Figure 2a).Signi cant interactions were
also present between KCl (B) and KH
2
PO
4
(C)with a low
p-value (0.0008) and between KH
2
PO
4
(C) and tannic
acid(A) with a low p-value (0.0085) (Figure 2b and 2c).
Validation of the Model: Validation of the response sur-
face model was con rmed by using opt imum conditions
obtained by the model. The experimental values were
very close to the predicted values and hence it was con-
cluded that the model was successfully validated.
CONCLUSION
The response surface methodology was applicable for
the production of tannase from Streptomyces sp. AT13.
In the present study,optimum conditions for tannase
production by Streptomyces sp. AT13 was done using
Plackett-Burmen design followed by Response surface
methodology using Box-Behnken design. From among
6 fermentative variables that were studied, 5 signi cant
variables were picked up by PBD. Among 5 variables
from PBD, 3 were further optimized by Box-Behnken
design. Analysis of variance (ANOVA) showed the sig-
ni cance of the model and the validity of the model was
con rmed by the veri cation experiments. P-value less
than 0.05 indicate that the model terms are signi cant.
The optimum values of parameters obtained through
RSM were 1 % tannic acid, 0.5 % KCl and 0.1% KH
2
PO
4
to achieve maximum tannase production.
ACKNOWLEDGMENTS
The Authors are thankful to the head of Department of
Biotechnology and Microbiology, KSV University for
providing all the laboratory facilities. The authors are
also thankful to Biogene department of Gujarat State
Biotechnology Mission (GSBTM), Gandhinagar, Gujarat,
India for bacterial strain identi cation.
COMPLIANCE WITH ETHICAL STANDARDS
Con ict of Interests: There are no con icts of interest.
REFERENCES
Barthomeuf, C., Regerat, F., Pourrat, H. (1994). Production,
puri cation and characterization of a tannase from Aspergil-
lus niger LCF 8. J. Ferment. Bioeng. 3: 320-323.
Bate-Smith, E.C. and Swain, T. (1962). Flavonoid compounds.,
In: Comparative biochemistry. Academic Press, Pp 755-809
(Eds) Mason and A.M. Florkin. New York.