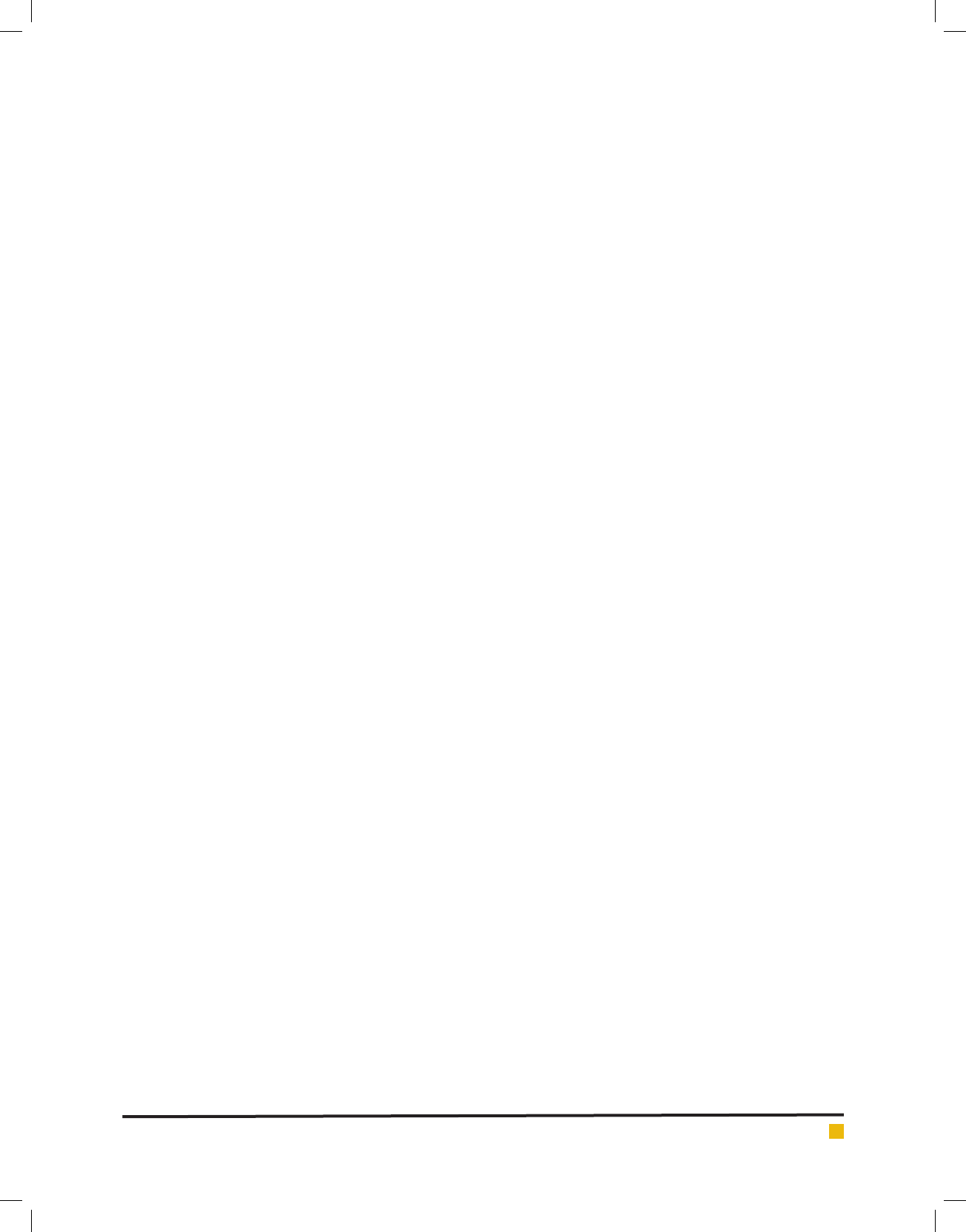
Sharareh, Aghafakhr and Ghodratollah
among GCA and MPH under drought stress conditions
is an indicator that GCA can be useful to predict MPH
during selections under water stress conditions.
REFERENCES
Amini, F., A. Mirlohi, M.M. Majidi, S. Shojaiefar, R. Kolliker
(2011) Improved polycross breeding of tall fescue through
marker-based parental selection. Plant Breeding 130: 701-
707.
Aminu, D., S.G. Mohammed, B.G. Kabir (2014) Estimates of
combining ability and heterosis for yield and yield traits in
maize population (Zea mays L.), under water conditions in the
Northern Guinea and Sudan Savanna zones of Borno State,
Nigeria. International Journal of Agriculture Innovations and
Research 2 (5): 824-830.
Amorim, E.P., V.B.O. Amorim, J.B. Dos Santos, A.P. De Souza,
J.C. De Souza (2006) Genetic distance based on SSR and grain
yield of inter and intra population maize single cross hybrids.
Maydica 51: 507–513.
Balestre, M., R.G. Von Pinho, J.C. Souza, J.L. Lima (2008) Com-
parison of maize similarity and dissimilarity genetic coef -
cients based on microsatellite markers. Genetics and Molecular
Research 7: 695–705.
Bernardo, R (1992) Relationship between single-cross perfor-
mance and molecular marker heterozygosity. Theoretical and
Applied Genetics 83: 628-634.
Clarke Topp, C., G.W. Parkin, T.P.A. Ferre (2007) Soil water
content. In: M.R. Carter, E.G. Gregorich, editors, Soil sampling
and methods of analysis. Canadian Soc. Soil Sci., Pinawa.
Devi, P., N.K. Singh (2011) Heterosis, molecular diversity, combin-
ing ability and their inter-relationships in short duration maize
(Zea mays L.) across the environments. Euphytica 178: 71–81.
Dhliwayo, T., K. Pixley, A. Menkir, M. Warbuton (2009) Com-
bining ability, genetic distances and heterosis among elite
CIMMYT and IITA tropical maize inbred lines. Crop Science
49: 1201–1210.
Falconer, D.S., T.F.C. Mackay (1996) Introduction to quantita-
tive genetics (4th ed.). Longman, London.
Frisch, M., A. Thiemann, J. Fu, T.A. Schrag, S. Scholten, A.E.
Melchinger (2010) Transcriptome-based distance measures for
grouping of germplasm and prediction of hybrid performance
in maize. Theoretical and Applied Genetics 120: 441 - 450.
George, M.L.C., F. Salazar, M. Warbuton, L. Narro, F.A. Vallejo
(2011) Genetic distance and hybrid value in tropical maize
under stress and non-stress conditions in acid soils. Euphytica
178: 99–109.
Hahn, V., K. Blankenhorn, M. Schwall, A.E. Melchinger (1995)
Relationships among early European maize inbreds. III. Genetic
diversity revealed with RAPD markers and comparison with
RFLP and pedigree data. Maydica 40: 299-310.
Hallauer, A.R., M.J. Carena, J.B. Miranda Filho (2010) Hand-
book of plant breeding: quantitative genetics in maize breed-
ing. Springer, New York.
Li, H., Q. Yang, N. Fan, M. Zhang, H. Zhai, Zh. Ni, Y. Zhang (2017)
Quantitative trait locus analysis of heterosis for plant height and
ear height in an elite maize hybrid zhengdan 958 by design III.
BMC Genetics 18: 36. DOI 10.1186/s12863-017-0503-9.
Linhart, Y.B., M.C. Grant (1996) Evolutionary signi cance of
local genetic differentiation in plants. Annual Review of Ecol-
ogy and Systematics 27: 237–277.
Maenhout, S., B.D. Baets, G. Haesaert (2010) Prediction
of maize single-cross hybrid performance: support vector
machine regression versus best linear prediction. Theoretical
and Applied Genetics 120: 415 - 427.
Mantel, N. A (1967) The detection of disease clustering and
a generalized regression approach. Cancer Research 27: 209-
220.
Melchinger, A.E (1999) Genetic diversity and heterosis. In:
J.G. Coors, S. Pandey, editors. The Genetics and Exploitation
of Heterosis in Crops, 99-118. ASA, CSSA and SSA, Madison,
WI, USA.
Mohammadi, S.A., B.M. Prasanna, C. Sudan, N.N. Singh (2008)
SSR heterogenic patterns of maize parental lines and predic-
tion of hybrid performance. Biotechnoloy & Biotechnoloical
Equipment 22 (1): 541-547.
Mohammed, W., D.P. Pant, Ch. Mohan, M. Arif Khan (2014)
Predicting heterosis and F1 performance based on combing
ability and molecular genetic distance of parental lines in Ethi-
opian mustard. East African Journal of Sciences 8 (2): 105-124.
Murray, M.G., W.F. Thompson (1980) Rapid isolation of high
molecular weight plant DNA. Nucleic Acids Research 8: 4321-
4325.
Ndhlela, T., L. Herselman, K. Semagn, C. Magorokosho, C.
Mutimaamba, M.T. Labuschagne (2015) Relationships between
heterosis, genetic distances and speci c combining ability
among CIMMYT and Zimbabwe developed maize inbred lines
under stress and optimal conditions. Euphytica 204: 635–647.
Pheirim, R., R. Niyaria, P. Kumar Singh (2017) Heterosis predic-
tion through molecular markers. Rising 1 (1): 45-50.
Prasanna, B.M. (2012) Diversity in global maize germ plasm:
characterization and utilization. Journal of Biosciences 37(5):
843–855.
Rohlf, F.J. (1998) NTSYS-pc numerical taxonomy and multi-
variate analysis system. Version 2.0. Exeter Software, Setauket,
NY.
SAS Institute. 2008. ‘User’s guide. Release 9.2.’ SAS Inst. Inc.,
Cary, NC.
Singh, R.K., B.D. Chaudhary (1977) Biometrical methods in
quantitative genetic analysis. Kalyani Publishers, New Delhi.
Srdic, J., S. Miladenovic-Drinic, Z. Pajic, M. Filipovic (2007)
Characterization of maize inbred lines based on molecular
markers, heterosis and pedigree data. Genetika 39: 355–363.
Steiner, J.J., G.G. Los Santos (2001) Adaptive ecology of Lotus
corniculatus L. genotypes: I. Plant morphology and RAPD
maker characterizations. Crop Science 41: 552–563.
BIOSCIENCE BIOTECHNOLOGY RESEARCH COMMUNICATIONS RELATIONSHIP AMONG COMBINING ABILITY, HETEROSIS AND GENETIC DISTANCE 223