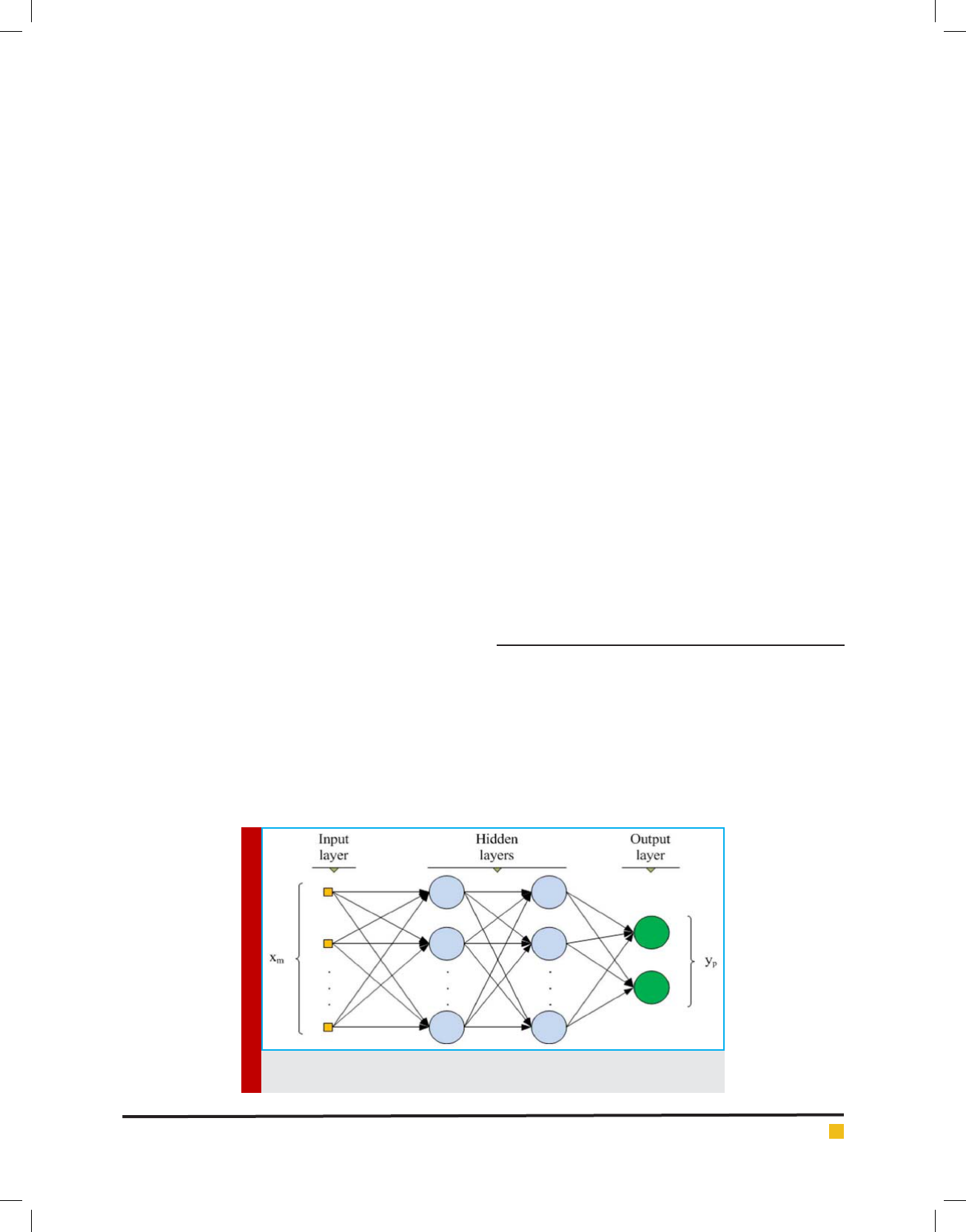
Goughari, Zayandehroodi and Eslami
BIOSCIENCE BIOTECHNOLOGY RESEARCH COMMUNICATIONS ENVIRONMENTAL ORIENTED OPTIMAL SELECTION OF RENEWABLE AND GREEN ENERGY SOURCES 331
Planning for energy supply for next generations is
one of the most crucial concerns of governments. The
planning calls for suitable estimation of power systems’
demands in future. The planning is performed in three
parts, i.e. load prediction, production planning, and
transmission planning. The most important part of plan-
ning in a power system is load prediction. Due to its high
capability in learning and relationship between inputs
and outputs, neural network is one of the most ef cient
methods.
Neural networks are inspired from information pro-
cessing from biologic neural system and processes
information as brain does. Key element of the idea is
the novel structure of information processing system;
the system is comprised of several processing elements
which coordinately work for problem solving (Passino,
2002).Like humans, Arti cial Neural Networks (ANNs)
learn by examples. An ANN is adjusted for performing
a known task such as recognition of patterns and clas-
si cation of data during a learning process. In biologic
systems, learning is accompanied by adjustments in
synaptic connections between nerves (Lakshminarasim-
man and Subramanian 2008).
The present work was formulated in order to obtain
proper amount of load needed in Bafgh for 2017. The
study was performed in ve sectors, i.e. industry, house-
hold, business, public, and agriculture. The estimation
was executed in order to prevent from occurrence of
troublesome conditions in network such as overload in
lines, network failure, etc. it is possible to increase con -
dence level of network in this city so that electrical sec-
tor’s authorities can have suitable programs in different
parts of electrical industry in Bafgh on the basis of the
results of the present work. After obtaining the results
relative to load prediction for Bafgh, optimization of a
compound generator system (wind and solar) independ-
ent of the network was considered for this city.
A technique for the optimal planning and design of
hybrid renewable energy systems for microgrid applica-
tions is presented in (Jung, et al. 2016); a novel approach
is used to determine the optimal size and type of dis-
tributed energy resources (DERs) and their operating
schedules for a sample utility distribution system. The 4
main aspects for energy ef ciency in a building consist
of rst the nearly zero energy passive building design
before actual construction, secondly the employment of
low energy building materials during its construction,
thirdly use of energy ef cient equipment for low oper-
ational energy requirement while lastly integration of
renewable energy technologies for various applications.
These aspects along with their economics and environ-
mental impacts are discussed by Chel, et al. (2017).
Recently, Hong, et al. (2017) have investigated the
optimal sizing of renewable energy generation resources
in a community microgrid; the cost of renewables and
community welfare are optimized while the comfort
zone of indoor temperature in all houses is maintained
using air conditioning systems. Similarly, Tezer, et al.
(2017) have also with the aim of investigating optimiza-
tion techniques, developed from past to present to solve
the problems of stand-alone hybrid renewable energy
systems and especially have tried to determine the ef -
cacy of multi-objective optimization approaches.
MATERIALS AND METHODS
Neural network estimations in SPSS
SPSS Software (Statistical Package for the Social Sci-
ences) can be adopted to run prediction through neu-
ral network imbedded inside the software. Here, weight
coef cient and role of each independent variable are
determined in prediction of dependent variable by using
toolbar of neural network (Chen, 2000).
FIGURE 1. Neural network structure for choosing optimal plants of distributed
production