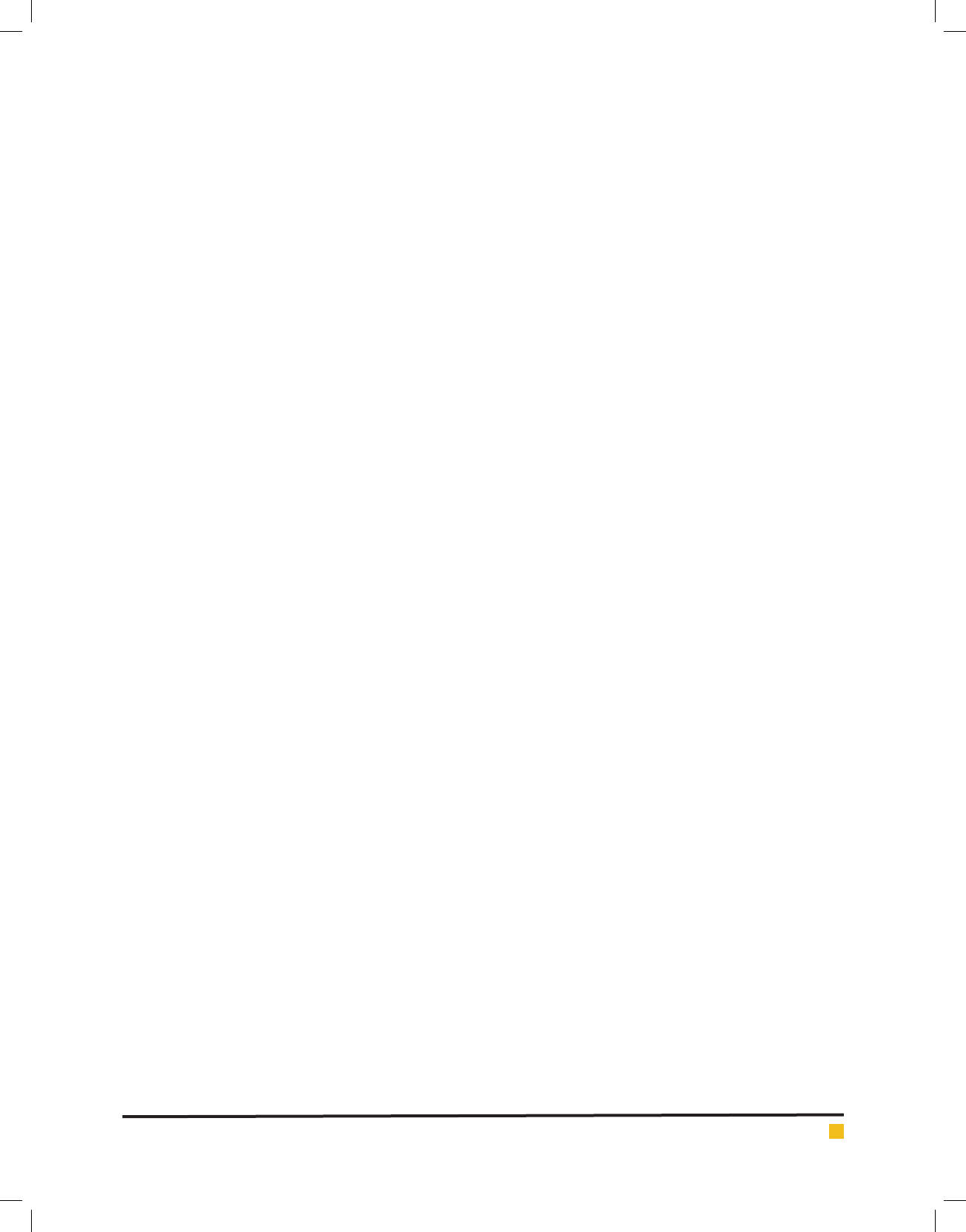
Prateek A. Meshram and Pradeep Singh
BIOSCIENCE BIOTECHNOLOGY RESEARCH COMMUNICATIONS AN EFFICIENT DENSITY-BASED ALGORITHM FOR CLUSTERING GENE EXPRESSIONS 31
Dettling, M. & Bühlmann, P., 2002. Supervised clustering of
genes. Genome biology, 3(12), p.RESEARCH0069.
Ester, M. et al., 1996. A Density-Based Algorithm for Discover-
ing Clusters in Large Spatial Databases with Noise. Proceed-
ings of the 2nd International Conference on Knowledge Dis-
covery and Data Mining, pp.226–231.
Frossyniotis, D., Likas, A. & Stafylopatis, A., 2004. A clustering
method based on boosting. Pattern Recognition Letters, 25(6),
pp.641–654.
Herrero, J., Valencia, a & Dopazo, J., 2001. A hierarchical unsu-
pervised growing neural network for clustering gene expression
patterns. Bioinformatics (Oxford, England), 17(2), pp.126–136.
Heyer, L.J., Kruglyak, S. & Yooseph, S., 1999. Exploring
Expression Data : Identi cation and Analysis of Coexpressed
Genes Exploring Expression Data : Identi cation and Analysis
of Coexpressed Genes. , (213), pp.1106–1115.
Huang, S. et al., 2015. Spectral co-clustering ensemble. Knowl-
edge-Based Systems, 84, pp.46–55. Available at: http://dx.doi.
org/10.1016/j.knosys.2015.03.027.
Jiang, D. & Zhang, A., 2003. DHC: a density-based hierarchical
clustering method for time series gene expression data. Third
IEEE Symposium on Bioinformatics and Bioengineering, 2003.
Proceedings., pp.393–400. Available at: http://ieeexplore.ieee.
org/lpdocs/epic03/wrapper.htm?arnumber=1188978.
Ka Yee Yeung, David R. Haynor, W.L.R., Validating Clustering
for Gene Expression Data. Available at: http://staff.washing-
ton.edu/kayee/cluster.
Li, X. & Tian, Z., 2007. Optimum cut-based clustering. Signal
Processing, 87(11), pp.2491–2502.
Lipkus, A.H., 1999. A proof of the triangle inequality for the
Tanimoto distance. , 26, pp.263–265.
Liu, Y. et al., 2014. A network-assisted co-clustering algorithm
to discover cancer subtypes based on gene expression. BMC
bioinformatics, 15(1), p.37. Available at: http://www.biomed-
central.com/1471-2105/15/37.
Marco Locatelli, Mirko Maischberger, and F.S., 2014. No Title.
Differential evolution methods based on local searches. Com-
puters & Operations Research, 43:169–180, 2014.
Meagher, R.B., 2002. Post-genomics Networking of Biotech-
nology for Interpreting Gene Function. Current Opinion in
Plant Biology, 5(2), pp.135–140. Available at: http://dx.doi.
org/10.1016/S1369-5266(02)00245-5.
Nakamura, E. & Kehtarnavaz, N., 1998. Determining num-
ber of clusters and prototype locations via multi-scale clus-
tering. Pattern Recognition Letters, 19(14), pp.1265–1283.
Available at: http://www.sciencedirect.com/science/article/pii/
S0167865598000993.
Nepomuceno, J.A., Troncoso, A. & Aguilar-Ruiz, J.S., 2011.
Biclustering of gene expression data by correlation-based scat-
ter search. BioData mining, 4(1), p.3. Available at: http://www.
pubmedcentral.nih.gov/articlerender.fcgi?artid=3037342&tool
=pmcentrez&rendertype=abstract.
Rand, W.M. & Rand, W.M., 2016. Objective Criteria for the
Evaluation of Clustering Methods Objective Criteria for the
Evaluation of Clustering Methods. , 66(336), pp.846–850.
Searls, D.B., 2000. Using Bioinformatics in Gene and Drug Dis-
covery. Drug Discovery Today, 5(4), pp.135–143.
Storn, R. & Price, K., 1997. Differential Evolution – A Simple
and Ef cient Heuristic for global Optimization over Continu-
ous Spaces. Journal of Global Optimization, 11(4), pp.341–359.
Available at: http://dx.doi.org/10.1023/A:1008202821328.
Tamayo, P. et al., 1999. Interpreting patterns of gene expres-
sion with self-organizing maps: methods and application to
hematopoietic differentiation. Proceedings of the National
Academy of Sciences of the United States of America, 96(6),
pp.2907–2912.
Wagner, S. & Wagner, D., 2007. Comparing Clusterings - An
Overview. , (1907), pp.1–19.
Wang, H., Zheng, H. & Azuaje, F., 2007. Poisson-based self-
organizing feature maps and hierarchical clustering for serial
analysis of gene expression data. IEEE/ACM transactions on
computational biology and bioinformatics / IEEE, ACM, 4(2),
pp.163–75. Available at: http://www.ncbi.nlm.nih.gov/pub-
med/17473311.
Xiao, X. et al., 2003. Gene clustering using self-organizing
maps and particle swarm optimization. Parallel and Distributed
Processing Symposium, 2003. Proceedings. International, 0(C),
p.10–pp. Available at: http://ieeexplore.ieee.org/xpls/abs_all.
jsp?arnumber=1213290.
Yang Lou, Junli Li, and Y.W., A Binary-Differential Evolution
algorithm based on Ordering of individuals. IEEE Natural Com-
putation (ICNC), 2010 Sixth International Conference on.
Yeung, K.Y. & Ruzzo, W.L., 2001. Principal component analy-
sis for clustering gene expression data. Bioinformatics, 17(9),
pp.763–774. Available at: http://www.cs.washington.
Yu, X., Yu, G. & Wang, J., 2017. Clustering cancer gene expres-
sion data by projective clustering ensemble. Plos One, 12(2),
p.e0171429. Available at: http://dx.plos.org/10.1371/journal.
pone.0171429.
Yu, Z. et al., 2013. for Tumor Clustering from Biomolecular
Data. , 10(3), pp.657–670.
Zaït, M. & Messatfa, H., 1997. A comparative study of cluster-
ing methods. Future Generation Computer Systems - Special
double issue on data mining, Volume 13(Issue 2-3), p.Pages
149-159.
Zou, D. et al., 2013. A modi ed differential evolution algo-
rithm for unconstrained optimization problems. Neurocomput-
ing, 120, pp.469–481.